Thank you for subscribing. About 50% of this post is available as a free preview. Occasional forwarding is okay. If you are not a member, please sign up here.
A country does not rise to the level of its innovation. It falls to the level of its industrial commons. - Real Charts
Diffusion Deficit
Bill Bishop recently introduced me to the concept of a diffusion deficit in a Substack note reply. (Side note: The quality of engagement and writing on Substack continues to impress me. It’s a pleasure to be here.)
The term comes from Jeffrey Ding’s paper, “The Diffusion Deficit in Scientific and Technological Power: Re-assessing China’s Rise.” His research challenges the conventional wisdom that China is poised to become a dominant science and technology superpower.
What is a Diffusion Deficit?
Ding’s work highlights that standard assessments of China’s technological progress—measured by patents, scientific papers, and R&D spending—fail to account for diffusion capacity, or how well a country scales and integrates innovations into its economy.
Ding argues that, much like the Soviet Union in the 1960s, China has a strong innovation capacity but a weaker diffusion capacity. While China excels at producing cutting-edge research and prototypes, its ability to scale and integrate these innovations across industries lags behind.
A diffusion-centered approach shifts the focus from mere invention to the adoption of innovation at scale throughout an economy, society or industry.
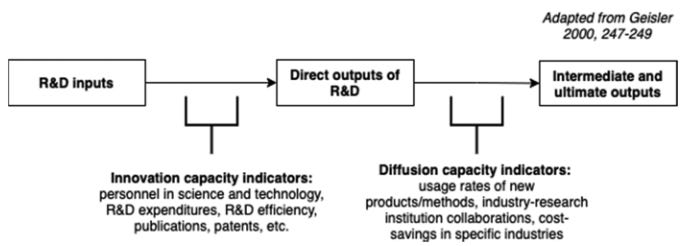
While Ding’s efforts to measure diffusion capacity are commendable—it’s an incredibly difficult task! Many of the metrics used are broad and survey-based or just plain estimates, raising questions about their accuracy.
One example is ITU’s ITC Development Index (see interactive data here)1, which measures 3G and 4G mobile network coverage but not 5G, which China leads. It also lists mobile phone owners at 100% in the U.S. but only 83% in China, despite:
China ranks number 1 in scale of mobile phone subscribers at 1.7 billion
First-hand observations of widespread mobile phone usage in China, by anyone who’s visited.
This discrepancy illustrates the challenge of relying on high-level diffusion indicators without considering industry-specific dynamics.
In my humble opinion, a true measure of diffusion capacity should assess the quality of the industrial commons. Other key factors would include the intensity of industrial competition, which drives firms into a trial-and-error process of turning innovations into efficient, mass-produced realities. This is where industrial commons and manufacturing clusters come into play—something China has in abundance.
In the following sections, I examine three key factors that influence diffusion:
Proximity in supply chains
Industrial robots as a flawed diffusion metric
Industry-academia collaboration
Proximity and Speed of Innovation
Diffusion often works best when suppliers and production lines are closely integrated. This creates a rapid feedback loop where manufacturing problems are identified and solved in real time. Consider this scenario:
Production line workers notice a flaw and tweak a part.
They walk it over to a nearby supplier—literally next door.
The supplier immediately adjusts their manufacturing process.
The new version is seamlessly implemented in future production.
This process accelerates problem-solving and ensures rapid iteration—one of the keys to successful adoption of new technology, or diffusion capacity.
High proximity is a core feature of a robust industrial commons: clustering of resources, suppliers and talent, a.k.a. manufacturing clusters.
China’s industrial commons are robust with strong regional clusters. State media (which I take with a grain of salt) likes to highlight this:
“As a key driver of high-quality manufacturing development, China has established 178 national high-tech zones and 45 national advanced manufacturing clusters, scattered throughout the country.” - Global Times2
Industrial Robots: A Misleading Diffusion Indicator?
Some claim China’s slow tech diffusion is evident in its industrial robot adoption rates. But let’s break this down:
China has installed 4.12 times more industrial robots than the U.S. since 2011 (chart above).
But China also has 10 times more factories (6.03 million3 vs. 605,5484 in the U.S.).
If you compare these figures, China has fewer robots per factory than the U.S.—which could be interpreted as a diffusion gap.
This reasoning misses the bigger picture. Why should we care how evenly distributed industrial robots are across factories? In manufacturing, scale and efficiency matter more than per-factory diffusion.
If China has more industrial robots and a strong industrial commons to produce them at scale, that advantage outweighs concerns about their relative spread.